Banking LLMs vs GPT-4: Making the Right Choice for BFSIs
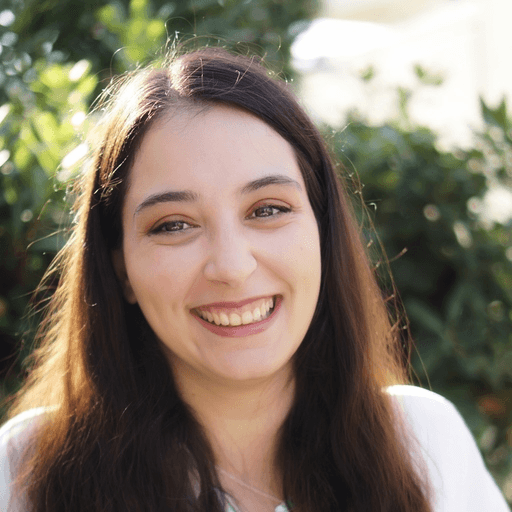
Panagiota
Product Marketing Manager
5 de fevereiro de 2025
in
🤖 Automação de IA
Financial services leaders face a crucial decision point in their technology adoption journey. 72% of financial firms have already integrated AI into their daily operations, highlighting the sector's rapid embrace of artificial intelligence. However, a critical decision emerges as financial institutions navigate the AI landscape: Should they opt for industry-specific Large Language Models (LLMs) trained exclusively on banking data or leverage general-purpose models like GPT-4 and, more recently, DeepSeek?
The real challenge lies in choosing and adopting the right solution for your needs. Let's dive into the key considerations.
Understanding Large Language Models
Large Language Models (LLMs) are sophisticated AI systems designed to understand, generate, and interact with human language. While general-purpose LLMs like GPT-4, Gemini, and DeepSeek have gathered significant attention, specialized Banking LLMs offer unique advantages for financial institutions.
Banking LLMs: Purpose-Built for Finance
Banking LLMs are specifically engineered for the financial sector, with deep domain expertise, regulatory compliance, and security built into their core architecture. These specialized models are trained extensively on financial data, regulations, and industry-specific terminology, enabling them to handle complex financial concepts with precision.
Here’s how they compare to GPT-4 across key areas:
Domain Expertise & Context Understanding
The financial services industry has highly specialized knowledge, complex regulatory frameworks, and intricate financial products. Understanding this domain requires more than just processing financial terms – it demands comprehending the interconnected nature of financial systems, regulatory implications, and market dynamics. This difference in domain expertise significantly impacts the models' ability to handle financial queries and operations effectively.
Banking LLMs
Trained extensively on financial data, regulations, and industry-specific terminology. This specialized training allows them to handle complex financial concepts with greater precision and understand nuanced banking terminology without additional context.
Pre-trained on vast amounts of financial documentation
Deep understanding of industry-specific terminology and concepts through specialized fine-tuning
Ability to interpret complex financial scenarios within proper regulatory frameworks
GPT-4
Foundational models like GPT-4, Gemini, etc., have been trained to create engaging conversations mainly for B2C scenarios. Therefore, while GPT-4 excels in general conversational AI applications, its broad training dataset lacks the deep financial expertise, regulatory understanding, and contextual awareness required for BFSI applications.
Broader knowledge base across multiple domains
May require additional context or prompting for complex financial tasks
Risk of misinterpreting specialized financial terminology
Regulatory Compliance & Data Security
In an era of increasing regulatory scrutiny and cybersecurity threats, financial institutions must prioritize compliance and data security. How an AI model handles sensitive financial information and adheres to regulatory requirements can significantly impact an organization's risk profile and compliance status.
Banking LLMs
These models are built with financial regulations in mind. They're typically pre-trained to understand GDPR, SOC-2, and other banking-specific compliance requirements, reducing the risk of non-compliant outputs.
Built-in compliance with financial regulations (GDPR, SOC-2, etc.)
Support for air-gapped, on-premise deployment
Encrypted data handling and processing
GPT-4
GPT-4 has been created as a “standalone” model and lacks built-in BFSI compliance. Institutions must add extra security layers to ensure regulatory adherence. Additionally, these models do not offer the on-premise deployment that large BFSIs often require.
Requires additional compliance layers
Limited deployment options
Higher risk of data exposure
Accuracy & Reliability
When dealing with financial transactions and advice, accuracy isn't just desirable – it's essential. The cost of errors in financial services can be substantial, both in terms of monetary impact and reputational damage. The reliability of AI responses in financial contexts directly affects operational efficiency and risk management.
Banking LLMs
Deliver precise responses to banking-related queries, minimizing hallucinations and misinformation.
Specialized training reduces hallucinations in financial contexts
Consistent responses aligned with banking policies
Lower risk of providing incorrect financial advice
GPT-4
While highly advanced, it may generate plausible but incorrect information, which can be risky in BFSI settings.
General-purpose accuracy across domains
Higher risk of hallucinations in financial scenarios
Less consistent in handling complex financial queries
Latency & Speed
In financial services, speed matters. Whether processing transactions, responding to customer queries, or analyzing market data, the ability to provide quick, accurate responses can provide a significant competitive advantage. The architectural differences between specialized and general-purpose LLMs directly impact their performance in time-sensitive financial operations.
Banking LLMs
Optimized for real-time financial interactions, ensuring low-latency responses.
Real-time response capabilities for critical operations
Can guarantee SLAs, especially if they are deployed in private instances
GPT-4
Computationally heavy, which may result in slower response times for complex queries.
Variable response time based on query complexity
May struggle with real-time financial operations
Resources intensive for large-scale deployments
Integration with BFSI Systems
Financial institutions operate with complex technology stacks, including core banking systems, trading platforms, and regulatory reporting tools. The ability to seamlessly integrate with these existing systems is crucial for maintaining operational efficiency and ensuring data consistency across platforms.
Banking LLMs
Seamlessly integrate with core banking platforms, CRMs, fraud detection systems, and regulatory reporting tools.
Native integration with core banking systems
Support for banking-specific APIs and protocols
Seamless integration with regulatory reporting tools
GPT-4
Requires additional customization and API integrations to connect with financial systems.
Requires custom integration development
Complex API mapping needs
Higher integration costs and complexity
Customization & Fine-tuning
Every financial institution has unique needs, products, and operating procedures. The ability to customize AI models to meet these specific requirements while maintaining security and compliance is crucial for successful implementation and adoption.
Banking LLMs
Pre-trained with BFSI-specific knowledge but customizable for institution-specific needs.
Lower resource requirements for customization
Targeted fine-tuning for specific banking needs
Faster adaptation to new financial products and processes
GPT-4
Customization is possible but requires extensive training to align with BFSI requirements.
Broad but shallow customization capabilities
Resource-intensive customization process
Higher data requirements for effective fine-tuning
Cost efficiency
While initial implementation costs are essential, the total cost of ownership, including ongoing operational expenses, customization needs, and scaling costs, should be carefully considered. The choice between specialized and general-purpose LLMs can significantly impact long-term financial outcomes.
Banking LLMs
More cost-effective in the long run as they require fewer modifications and optimizations.
Lower long-term operational costs
Efficient resources allocation
Lower training and fine-tuning expenses
GPT-4
High processing costs, especially for large-scale financial deployments.
Higher per-token costs
Additional costs for financial specialization
Higher infrastructure requirements

Choosing the Right AI for Your BFSI Institution
While GPT-4 is a powerful AI model, it is not inherently designed to meet the complex requirements of BFSI institutions. Banking LLMs offer clear advantages in domain expertise, compliance, security, accuracy, and integration, making them the superior choice for a robust conversational AI strategy.
At Moveo.AI, we specialize in proactive AI agents powered by Banking LLMs, enabling financial institutions to transform customer interactions. Our AI-driven solution is designed to go beyond basic chatbot responses—anticipating customer needs, ensuring compliance, and delivering real-time, personalized banking experiences.
The future of AI in BFSI isn’t just about automation—it’s about creating intelligent, seamless, and proactive customer engagement. Choosing the right AI model is a strategic decision, and for financial institutions looking to stay ahead, banking LLMs are the key to unlocking unparalleled efficiency and customer satisfaction.
Want to see Banking LLMs in action? Book a demo with Moveo.AI today.