Why GPT-5 won’t be enough to deploy AI Agents?
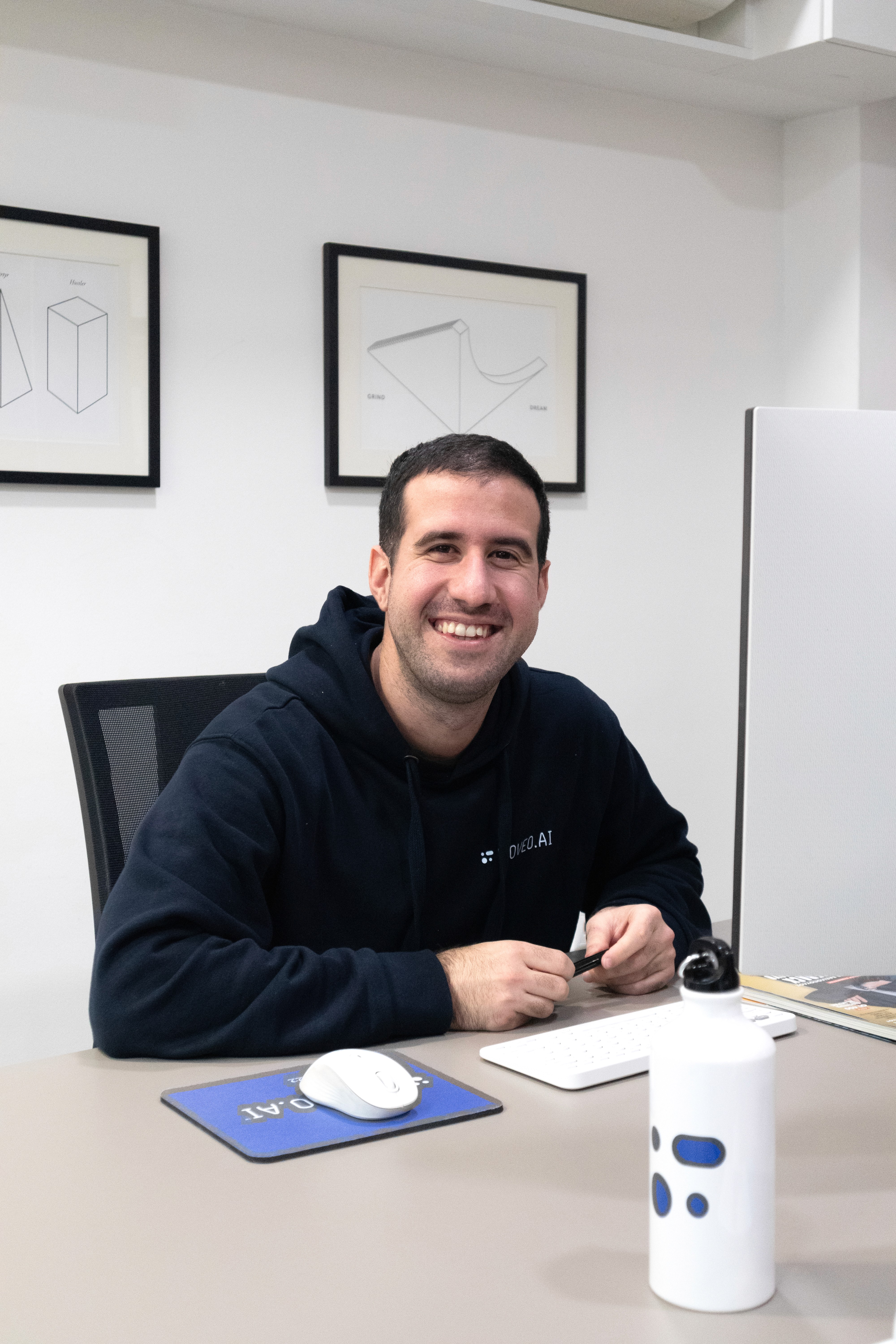
Panos
Co-founder & CEO
December 6, 2024
in
🏆 Leadership Insights
A common and reasonable question is: Why invest in building complex AI Agent architectures if future versions of GPT might eventually include all the necessary functionality out of the box?
Why Not Wait for GPT-5 to Handle It All?
The answer lies in the observation that progress in transformer architectures—the neural network type behind large language models—appears to be slowing down. Looking at benchmarks designed to evaluate the performance of LLMs, such as the Massive Multitask Language Understanding (MMLU), we observe a noticeable plateau in recent advancements. GPT-4 set a record in 2023 with an impressive 86.4% score, nearly doubling GPT-3's performance from its debut in 2020. However, since GPT-4's release, newer models have shown only marginal improvements compared to the significant leap from GPT-3 to GPT-4. For example, GPT-o1, OpenAI's latest reasoning model, scores around 92.3% on MMLU , only 6% increase over to GPT-4’s 86.4%. This suggests that while advancements continue, the transformative breakthroughs that defined earlier iterations are becoming harder to achieve.
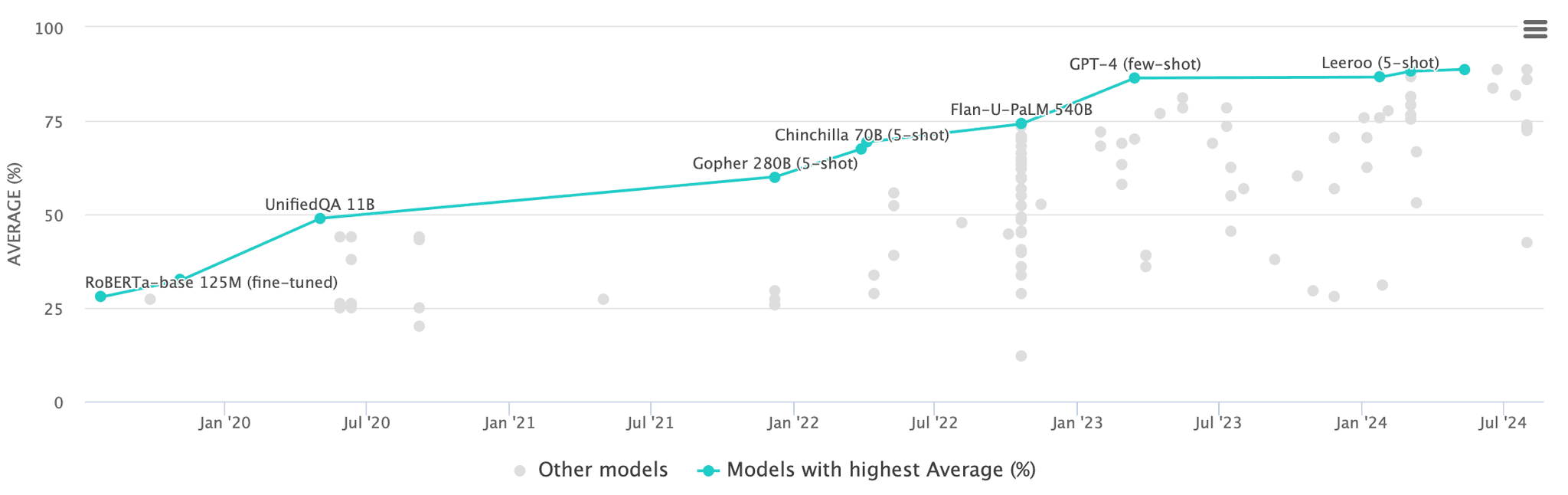
One of they key reasons why newer models exhibit only marginal improvements can be found by looking at a recent publication, titled No “Zero-Shot” Without Exponential Data. The paper exhibits evidence that additional training data provide diminishing returns in LLM performance improvements which exhibits a logarithmic trend as data increases.
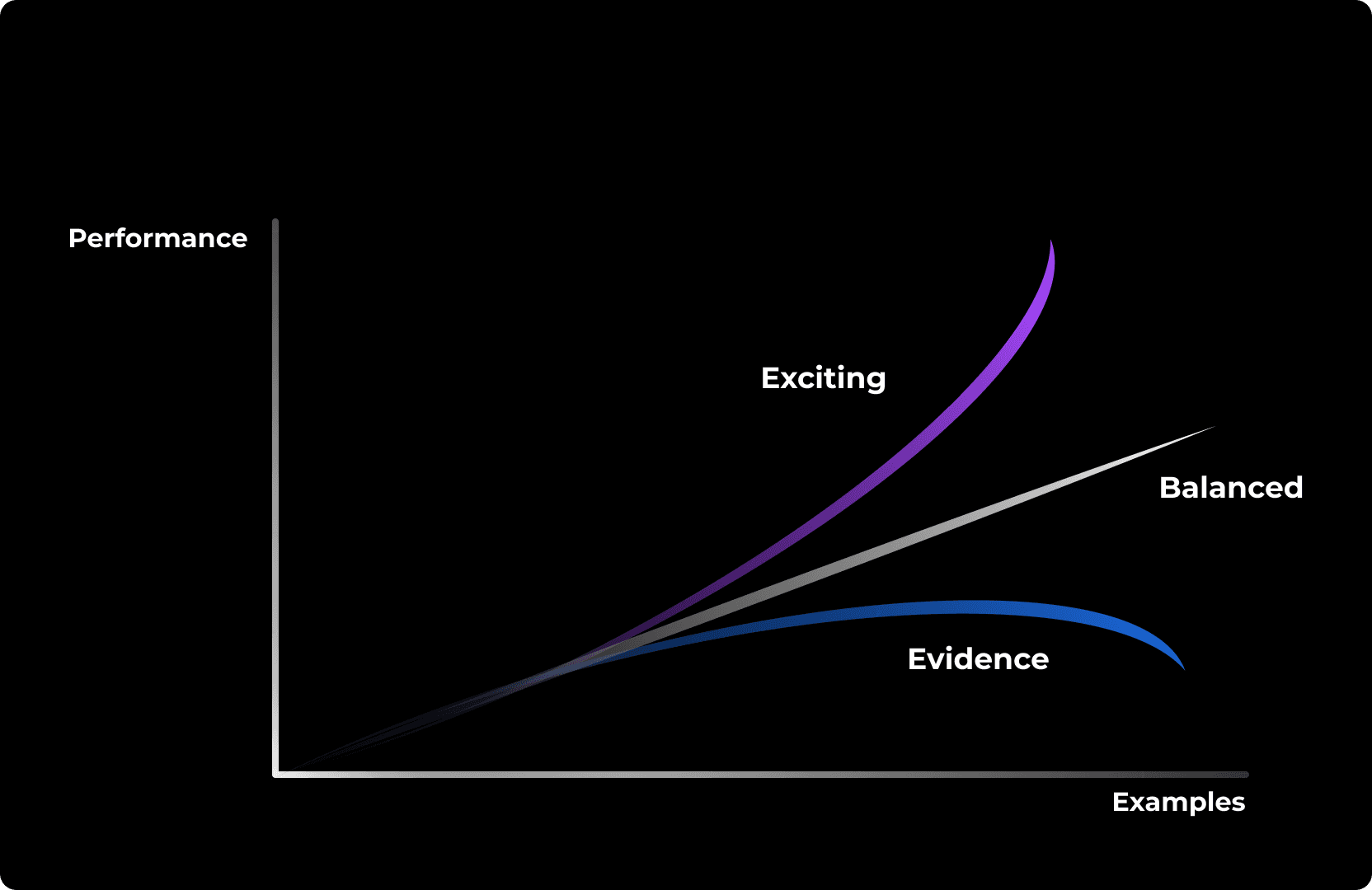
If this trend holds true as the evidence in the paper suggest, then we are faced with a situation where LLMs will need exponential more data in order to improve towards reaching AGI (Artificial General Intelligence). The issue is compounded by the fact that at approximately 15 trillion tokens, current LLM training sets are already approaching the upper limit of high-quality public text available. For English alone, estimates suggest a maximum range of 40–90 trillion tokens, meaning we are nearing the saturation point of usable and available data.
Moreover, historical trends indicate that model data requirements have increased tenfold with each new generation (GPT-2 to GPT-3 to GPT-4 all required 10x or more data). While GPT-5 might still achieve incremental improvements through expanded data collection and minor optimizations, scaling alone is unlikely to sustain the same trajectory for future generations. For models at the GPT-6 level and beyond, achieving meaningful progress will likely require breakthroughs in novel architectures or entirely new paradigms that have yet to be discovered.
Given the current state of research and available evidence, it is far from certain that future models like GPT-5 or GPT-6 will deliver improvements on the same order of magnitude as their predecessors. The argument that "it took three years for GPT-4 to surpass GPT-3" is weak when scrutinized: GPT-4’s leap in performance was primarily driven by scaling—larger architectures and 10x more training data.
This approach, however, not only faces diminishing returns—as evidence suggests—but we are also approaching the practical limits of available high-quality text data. Further progress will depend less on simply scaling up and more on innovation in new architectures or entirely different training paradigms; without such breakthroughs, the rapid advances we've witnessed in recent years may inevitably slow down. A counter-argument is that there is still an abundance of non-textual data, such as images and videos, which are rich sources of information. Indeed, a significant portion of human cognition comes from observing situations visually rather than through text. While models that process pictures and videos are already being developed and multimodal models are emerging, these technologies are still in their early stages—especially in the realm of video generation. Researchers in large R&D departments, are attempting to leverage this untapped data by creating models capable of recognizing situations in videos and images, converting them into text, and thereby enriching LLMs. While this holds promise, it underscores the need for innovative approaches rather than relying solely on scaling existing models.