Harnessing Conversational AI Analytics for CX excellence
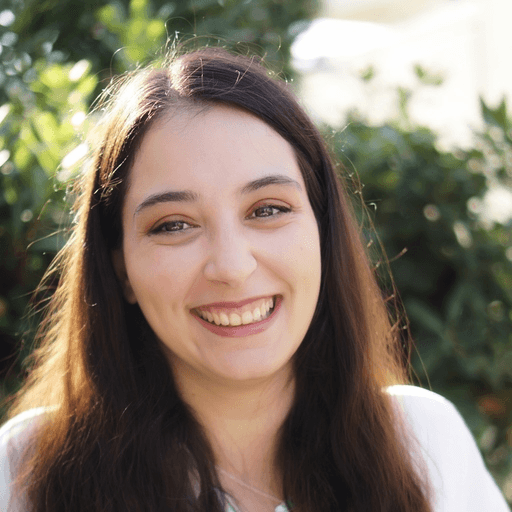
Panagiota
Marketing Specialist
February 8, 2024
in
👨👩👦 CX
Have you ever wondered about the conversations your business has with its customers? The insights they hold? Or the value they add to your analytics?
Digitalization is everywhere and the corporate world is no exception; we rely heavily towards data-driven decisions and automated customer service. According to Gartner, “Leading organizations in every industry are wielding data and analytics as competitive weapons”.
Let’s explore how Conversational AI analytics can shape CX excellence.
Conversational AI Analytics 101
Conversational AI Analytics is a technology that utilizes Machine Learning and Natural Language Processing (NLP) to analyze and understand customer interactions, usually in the format of voice or text conversations.
This powerful technology offers significant insights into customer behavior, preferences and satisfaction, paving the way for businesses to improve their services and provide a seamless, personalized, and engaging customer experience. These conversational insights are also essential to measure the efficiency of AI or live agents, guiding potential improvements based on user engagement and feedback.
The power of conversational insights not only enhances the customer experience, but also reveals hidden knowledge that can be crucial to product development, marketing strategy, and customer retention initiatives.
Benefits of Conversational AI analytics
Conversational AI Analytics offer several benefits in enhancing customer experiences (CX). Kaizen Gaming, for instance, harnesses Moveo’s conversational AI agents to streamline their customer service. Previously, this was solely handled by customer care representatives who manually sifted through customer feedback data. Now, their AI system reviews the data, discerns patterns, and offers quick solutions tailored to each customer issue, thus enhancing customer satisfaction (CSAT) and reducing response time.
Read How Kaizen Gaming experienced an 80% cost reduction in customer support operations.
Let’s dive into the benefits of Conversational AI analytics:
Deep Customer Insights
Understand your customer needs, wants and sentiments on a deeper level
Conversational AI allows for customization in discussions with customers, providing a personal touch. Specialized AI algorithms can analyze a large amount of customer conversations and draw meaningful, otherwise hidden, insights concerning customer behaviors, preferences, pain points as well as common mistakes or inappropriate behaviors by the human or AI agents. New AI algorithms based on Large Language Models (LLMs) can extract and report insights that would be otherwise impossible to track. Here are some examples:
– Agent politeness
– User sentiment change during the conversation
– Most common topics
– Adherence to data/security policies by the Agent, e.g “was the customer properly verified, before the agent gives out personal/sensitive information?”Informative Business Decisions
Tackle complex challenges and strategic decisions with the power of data-backed insights
– Real-time insights
With AI analytics, businesses can gain real-time insights into customer behavior, which can enable them to adjust their strategies quickly, if needed. This increases the ability to respond swiftly to market trends and changes. Let’s assume that a customer raises inquiries or expresses concerns regarding pricing. The AI agent can identify them as potential churn risks and seamlessly transfer the conversation to a live agent to prevent customer churn.
– Predictive Analytics
Utilizing conversational AI analytics provides a basis for predictive modeling. Businesses can forecast trends, anticipate customer needs, and make informed decisions based on patterns identified in large datasets.
By consistently analyzing and improving AI responses and engaging customers effectively, conversational AI analytics proves to be a valuable tool to enhance the customer journey, leading to increased customer satisfaction and ultimately, business growth.
The top KPIs in Conversational Analytics
Total conversations
This metric serves as a key performance indicator (KPI) to gauge the usage and popularity of your AI virtual agent. Monitoring this metric helps you understand the level of engagement and interaction between your customers and the AI agent.Conversations per channel and time of day
These metrics are crucial for understanding and optimizing customer engagement. By tracking these KPIs, businesses can not only understand which channels are most popular among their customers, but also schedule their resources efficiently during periods of high activity.Coverage and containment
These KPIs are used to evaluate the effectiveness of an AI virtual agent, particularly in the context of handling conversations. Coverage refers to the AI agent’s ability to address and respond to queries end-to-end. Containment, on the other hand, measures the conversations effectively managed by your AI agent without human intervention.Average response time and Resolution time
These KPIs are important in measuring the efficiency and performance of your support operations. They reflect the speed at which issues are addressed and successfully resolved.Customer satisfaction (CSAT)
CSAT is a pivotal metric in measuring the overall customer experience (CX). It provides valuable insights into the degree of contentment among your customers, indicating whether their needs were met and if they successfully found solutions to their queries or concerns.
Conclusion
Assessing AI performance and personalizing customer engagement using first-party data are all part of the powerful tool kit Conversational AI analytics offers. This transformation impels businesses to be proactive and responsive, maintaining a competitive edge in today’s digital marketplace.
Just remember, at the heart of your analytics should always be your customers – Conversational AI analytics merely opens the door for you to understand them better. Step through it and embrace this invaluable tool. The language of your success might just lie in the conversations you’re already having!